The new Llama 4 Scout and Maverick models boast massive context windows (the amount of text a model can process in a single go), native vision capabilities, and performance that positions them to rival open source systems like DeepSeek.
Faster and smarter than previous generations, these models are trained for enhanced reasoning, coding, and conversation — powered by a new Mixture of Experts (MoE) architecture, where multiple specialised sub-models collaborate dynamically to generate a response.
Meet the new herd
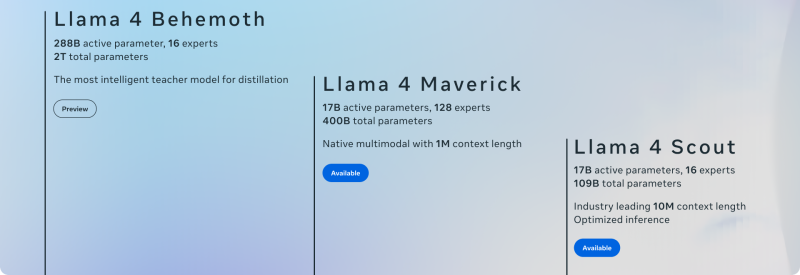
Meta’s Llama 4 family kicks off with two openly available models — Scout and Maverick — and a third, more powerful research model called Behemoth, currently in preview.
Llama 4 Scout is designed for accessibility and efficiency, packing 17 billion parameters across 16 experts.
It’s the smallest of the new herd, but it’s considerably larger than the small-scale versions from the prior generation, Llama 3.2.
Despite being bigger, though, Scout is capable of running on a single Nvidia H100 GPU when quantised — even then, it can handle 10 million tokens of context, making it ideal for long document summarisation, codebase analysis, and multi-modal input at scale
The middle version is Maverick, which stands at 17 billion active parameters and 128 experts.
Like Scout, the mid-tier Llama 4 model can run on a single H100 host and is Meta’s new workhorse for general-purpose AI tasks, from advanced reasoning to creative writing and multimodal chat.
Then there’s Llama 4 Behemoth — the largest and most capable model in the lineup, with 288 billion active parameters and nearly two trillion total parameters.
While not yet released, Behemoth is already a step up from the giant Llama 3.1 model released last July.
“Codistillation from Llama 4 Behemoth during pre-training amortises the computational cost of resource-intensive forward passes needed to compute the targets for distillation for the majority of the training data used in student training,” Meta’s blog post reads. “For additional new data incorporated in student training, we ran forward passes on the Behemoth model to create distillation targets.”
In simple terms, Meta used its powerful Llama 4 model as a teacher to help train the smaller Scout and Maverick models, allowing them to achieve impressive performance that rivals much larger AI systems while using fewer computing resources.
It was used to distil both Scout and Maverick, helping them achieve performance levels that compete with or exceed the likes of GPT-4.5, Claude 3 Sonnet, and Gemini 2.0 Pro on coding, STEM, and multilingual benchmarks despite being vastly smaller than rival systems.
Llama 4 Behemoth boasts its own impressive abilities, outperforming flagship models from OpenAI, Anthropic, and Google on industry standard benchmarks, including MMLU Pro, MMU, and GPQA Diamond.
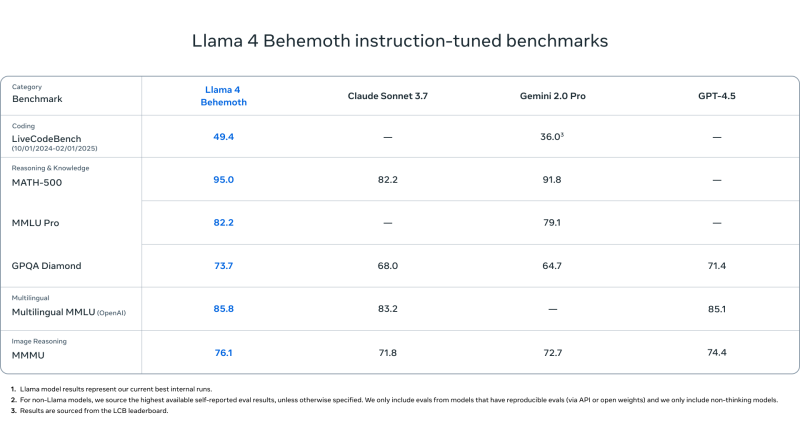
Multimodality by design
With Llama 4, Meta isn’t just scaling up, it’s building smarter, more flexible open source models from the ground up that can do more.
At the heart of its new herd is native multimodality: the ability to understand and reason across both text and visual inputs within the same model, without needing external adapters or separate components.
Unlike previous models that were “patched” to handle things like vision, Llama 4 is multimodal by design.
The models use what Meta calls early fusion, a technique that allows text and vision tokens to be processed in a shared model backbone from the start. Such a design enables Llama 4 models to better understand the relationship between words and images, whether it's answering questions about a picture, grounding visual references in language, or parsing sequences of visual data.
To power its vision capabilities, Llama 4 includes an upgraded MetaCLIP-based vision encoder — essentially, it's trained alongside a frozen language model to optimise how images are interpreted in context.
During pretraining, the models were shown up to 48 images at once, and during post-training, they performed well with up to 8 images in tasks that involved image reasoning and visual question answering.
The result is a set of models that can handle complex visual inputs just as confidently as they handle language.
Llama 4 Scout, for example, excels at image grounding, aligning user prompts with visual elements to localise and reference objects more precisely — useful for tasks like identifying faults in network diagrams, annotating satellite images, or tagging infrastructure components in site photos.
Under the hood: MoE, scaling smarts
The standout shift in Llama 4’s design is its move to a Mixture of Experts (MoE) architecture, a system that allows different parts of the model to specialise in different tasks, improving both efficiency and performance.
Rather than activating every parameter in the network for each input, MoE models only activate a subset of specialised “experts” depending on the task at hand. That means more compute can be allocated where it's needed, like handling a tricky coding query or analysing visual inputs, without bloating inference costs.
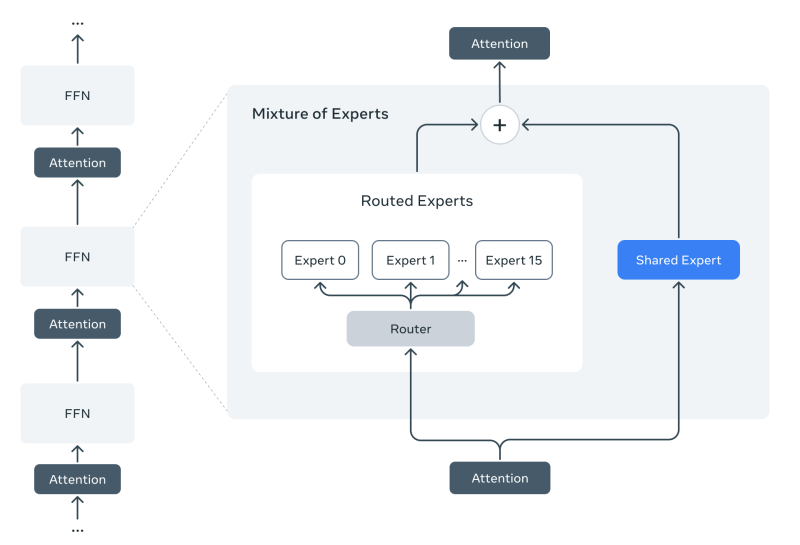
Llama 4 Scout uses 16 experts, while Maverick scales this up to 128, giving it more headroom to tackle complex multimodal or reasoning tasks. Importantly, even with large parameter counts, only 17 billion active parameters are used at any one time — keeping the models efficient enough to run on a single GPU.
Meta’s new hybrid approach — alternating dense and MoE layers — is key to the models’ ability to match or beat much larger systems like Gemini 2.0 and GPT-4.5, while also making them more practical to deploy across enterprise and developer environments.
Safety, bias, and governance
Meta is touting its new Llama models as being safer than ever, with the new generation developed with a layered safety strategy that spans pre-training, post-training, and system-level safeguards.
Pre-training datasets used to create Llama 4 models were filtered to remove harmful or low-quality content. Meanwhile, during post-training, Meta applied a range of reinforcement learning techniques to ensure the models behave reliably and helpfully.
Among the most visible safety layers are tools Meta has already offered to developers: Llama Guard (for flagging risky outputs), and Prompt Guard (to detect prompt injection and jailbreaks).
A new safety tool introduced alongside Llama 4 was the aptly named GOAT, or Generative Offensive Agent Testing, a red-teaming system that simulates adversarial interactions to test the model’s limits.
Where to access Llama 4
If you want to get your hands on the new herd, there are already several routes available.
Llama 4 Scout and Llama 4 Maverick are open-weight models — meaning you can download and run them yourself. They’re available now via llama.com, Hugging Face, and will soon roll out to major cloud platforms and integration partners.
Meta has also begun rolling out Llama 4 inside its own products, including Meta AI in WhatsApp, Messenger, Instagram, and on the Meta.AI website.
More insights are expected at LlamaCon on April 29, where Meta plans to share its broader roadmap, including where the ecosystem goes next and how developers across industries can build with Llama 4 at scale.
RELATED STORIES
Meta makes Llama AI models available to US gov, defence agencies
Meta unveils Llama 3.2: Smaller AI models for edge and mobile devices
Meta’s AI chief: DeepSeek proves AI progress isn’t about chips